Unlocking the Future: The Role of Medical Datasets for Machine Learning in Healthcare
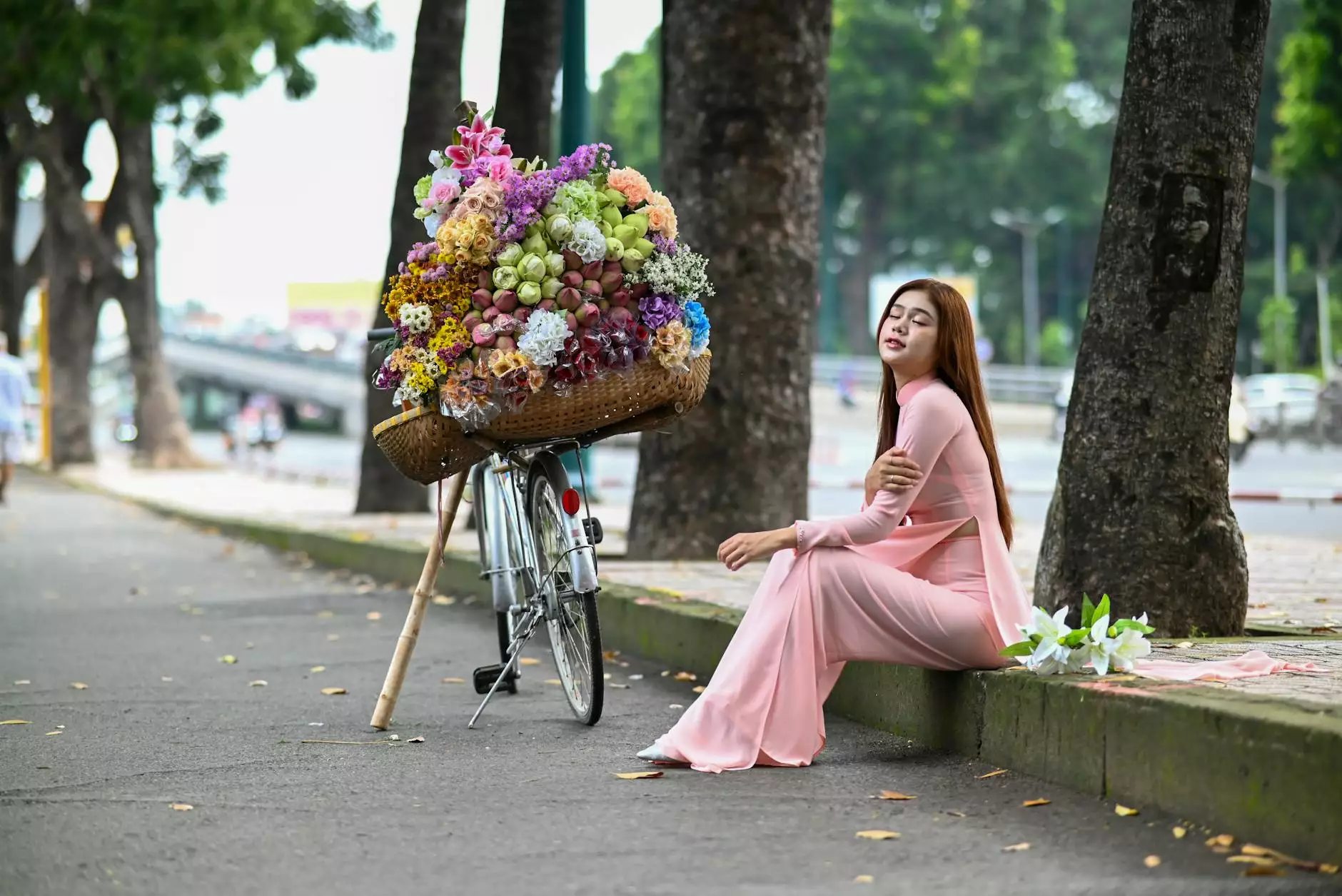
The integration of machine learning into healthcare has significantly transformed the way we approach medical research, diagnostics, and patient care. One of the cornerstones of this transformation is the availability and utilization of medical datasets for machine learning. These datasets empower researchers and healthcare professionals to develop predictive models that enhance outcomes, streamline operations, and ultimately improve patient safety. In this article, we will delve deep into the significance of these datasets, their applications, and the challenges faced in harnessing their full potential.
The Importance of Medical Datasets in Machine Learning
Machine learning thrives on data. In healthcare, where patient information is vast and varied, medical datasets for machine learning serve as the fuel for developing intelligent algorithms. The intricate nature of healthcare data makes it essential for researchers to have access to high-quality datasets that are both comprehensive and representative. These datasets can include:
- Electronic Health Records (EHRs): Rich repositories of patient histories, treatments, and outcomes.
- Clinical Trials Data: Results from clinical studies that provide insights into drug efficacy and safety.
- Genomic Data: Information on genetic variations that can affect health and treatment responses.
- Medical Imaging: Datasets consisting of X-rays, MRIs, and CT scans used for diagnosis.
- Wearable Device Data: Real-time health monitoring data from devices like fitness trackers and smartwatches.
Applications of Medical Datasets in Machine Learning
Understanding the applications of medical datasets for machine learning highlights their profound impact on various aspects of healthcare:
1. Predictive Analytics
One of the most significant advantages of utilizing machine learning in healthcare is the ability to perform predictive analytics. By employing historical data, healthcare providers can predict future events, such as hospital readmissions, disease outbreaks, or patient deterioration. For example:
- Predicting Patient Outcomes: Machine learning models can analyze EHRs to forecast how specific patients might respond to treatments, enabling personalized care.
- Resource Allocation: Using past admission data, hospitals can predict peak times, ensuring adequate staffing and resources are available.
2. Personalized Medicine
With a wealth of data available, machine learning can tailor treatments to individual patients. By processing genomic and clinical data, algorithms can identify the most effective therapies for patients based on their unique profiles. This approach can reduce trial-and-error in medication prescriptions and improve treatment outcomes.
3. Drug Discovery and Development
Machine learning significantly accelerates the drug discovery process. By analyzing vast datasets, researchers can identify potential drug candidates and predict their interactions with human targets. This not only shortens development timelines but also reduces costs associated with bringing new medications to market.
4. Medical Imaging Analysis
The use of machine learning algorithms to analyze medical images is revolutionizing diagnostics. Automated systems can detect anomalies in images faster and with greater accuracy than traditional methods. This aids radiologists by highlighting potential issues, ensuring no critical detail is overlooked.
5. Enhancing Operational Efficiency
Healthcare institutions can also utilize machine learning to optimize their operations. By analyzing patient flow and resource utilization, organizations can streamline processes, reducing wait times and improving patient satisfaction.
Challenges in Utilizing Medical Datasets for Machine Learning
While the advantages of medical datasets for machine learning are substantial, challenges remain that can impede their effective use:
1. Data Privacy and Security
With the increasing emphasis on data-driven decision-making, the protection of patient confidentiality is paramount. Healthcare organizations must navigate the complexities of regulations such as HIPAA in the United States. Ensuring that machine learning practices comply with such regulations while still allowing access to valuable datasets presents a significant challenge.
2. Data Quality and Standardization
The quality of machine learning outcomes is heavily dependent on the quality of data fed into algorithms. Variability in data formats, inconsistencies in data entry, and missing values can compromise the accuracy of predictions. Establishing standardized protocols for data collection and management is crucial for ensuring data integrity.
3. Interdisciplinary Collaboration
Successfully deploying machine learning in healthcare requires collaboration between data scientists and healthcare professionals. Bridging the gap between technology and clinical practice can sometimes be a hurdle, as these two fields often speak different languages. Continuous dialogue and education are essential for fostering effective interdisciplinary partnerships.
The Future of Medical Datasets and Machine Learning
The potential of medical datasets for machine learning in the healthcare sector is tremendously promising. As technology continues to evolve, we can anticipate the following trends:
1. Enhanced Interoperability
Future advancements are likely to focus on creating systems that enable different healthcare technology solutions to communicate and share data seamlessly. This interoperability will enhance access to comprehensive datasets, fueling improved machine learning models.
2. Advancements in Natural Language Processing (NLP)
As more unstructured data (like physician notes) is analyzed, NLP technologies will improve, allowing for better extraction of relevant clinical information. This ability will further enrich medical datasets, providing a more holistic view of patient health.
3. Focus on Equity in Healthcare
As machine learning continues to revolutionize healthcare, there will be a growing emphasis on ensuring that datasets represent diverse populations. Addressing disparities in healthcare data will lead to fairer outcomes and improved healthcare strategies for all demographic groups.
Conclusion
In conclusion, medical datasets for machine learning are not merely useful; they are essential in reshaping the landscape of healthcare. By leveraging these datasets, healthcare professionals can enhance patient care, streamline operations, and drive innovation in medical research. However, to truly harness the full power of this technology, it is vital to overcome the existing challenges and foster a collaborative environment between technology and healthcare practitioners. The future holds immense potential, and with continued efforts, we can unlock transformative advancements in healthcare through the effective use of machine learning.
For those in the healthcare industry, the time to embrace medical datasets for machine learning is now. By investing in data quality, focusing on ethical practices, and fostering collaboration, we can pave the way for breakthroughs that will benefit patients and healthcare systems worldwide.
medical dataset for machine learning